In the world of data-driven decision-making, Artificial Intelligence (AI) has become essential for deriving insights from massive datasets. AI-driven data analysis tools leverage machine learning, natural language processing, and advanced statistical techniques to uncover patterns, forecast trends, and optimize business operations. This article explores some of the top AI tools for data analysis, highlighting their features, use cases, and the unique advantages they bring to various industries.
Introduction to AI in Data Analysis
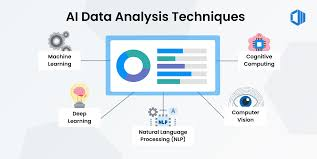
Why Use AI for Data Analysis?
- Automation of Manual Processes: Automates repetitive tasks, making analysis faster and reducing human error.
- Improved Predictive Analytics: Enhances forecasting accuracy for sales, demand, and risk assessment.
- Complex Pattern Detection: Identifies patterns not easily visible through traditional analysis methods.
Common Applications Across Industries
- Healthcare: AI for predictive diagnoses and personalized treatment plans.
- Finance: Fraud detection and automated risk management.
- Retail: Customer behavior prediction and supply chain optimization.
- Manufacturing: Process optimization and predictive maintenance.
Top AI Tools for Data Analysis
Tableau
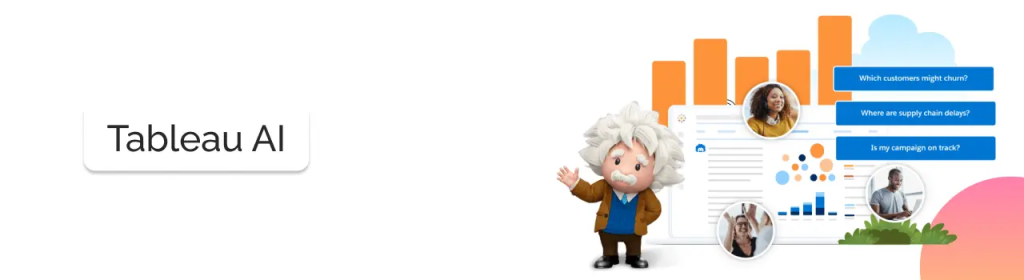
- Overview: Tableau is a data visualization tool enhanced by AI capabilities, making it easier to interpret complex datasets through visual storytelling.
- Features:
- AI-driven insights through natural language queries.
- Predictive analytics and trend analysis.
- Robust data integration and collaboration.
- Use Cases:
- Marketing analytics for tracking campaign performance.
- Financial forecasting and reporting.
- Why It Stands Out: Offers ease of use with advanced AI integration, making it accessible to non-technical users for data-driven decision-making.
Google Analytics 360 + BigQuery
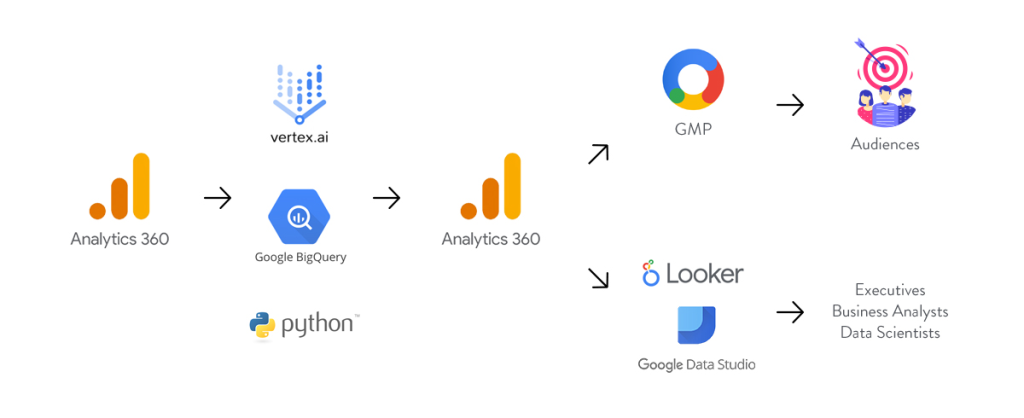
- Overview: Google Analytics 360, paired with BigQuery, brings advanced AI and machine learning capabilities for web analytics, especially useful for large-scale enterprises.
- Features:
- Predictive audience segmentation.
- Machine learning for churn prediction.
- Customizable dashboards for real-time insights.
- Use Cases:
- E-commerce analytics to enhance user engagement.
- Ad campaign optimization for better ROI.
- Why It Stands Out: Google’s ecosystem allows seamless integration with other Google products, bringing powerful, real-time analytics to marketing and customer experience teams.
Microsoft Power BI
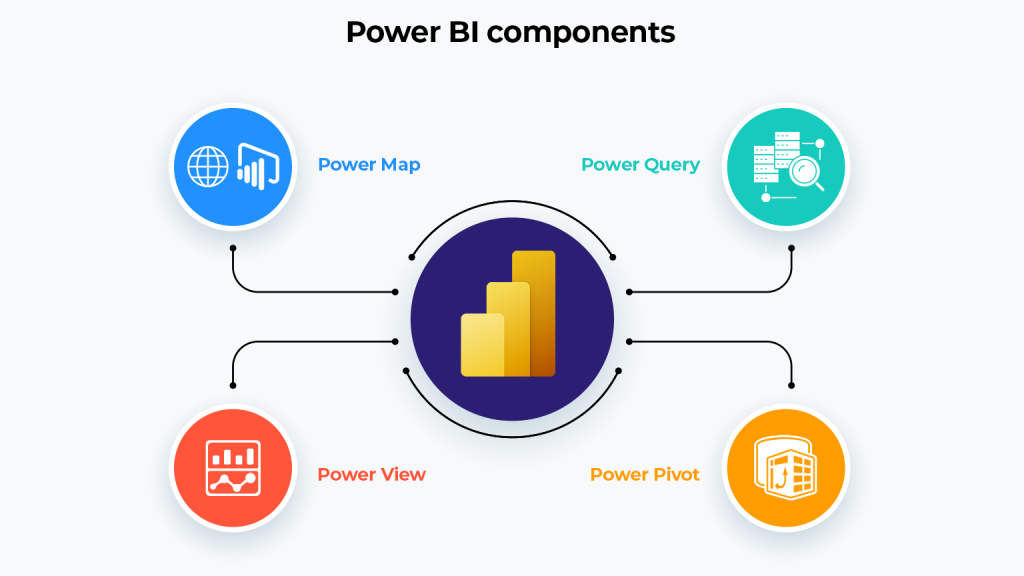
- Overview: Power BI combines data visualization with machine learning, enabling in-depth insights and AI-driven predictions.
- Features:
- AI-driven insights with natural language processing.
- Embedded machine learning models.
- Data integration from over 200 sources.
- Use Cases:
- Sales forecasting for businesses of all sizes.
- Performance analytics for departments within organizations.
- Why It Stands Out: Power BI is highly customizable and scalable, making it suitable for organizations that require specific data insights and seamless integration with Microsoft products.
IBM Watson Analytics
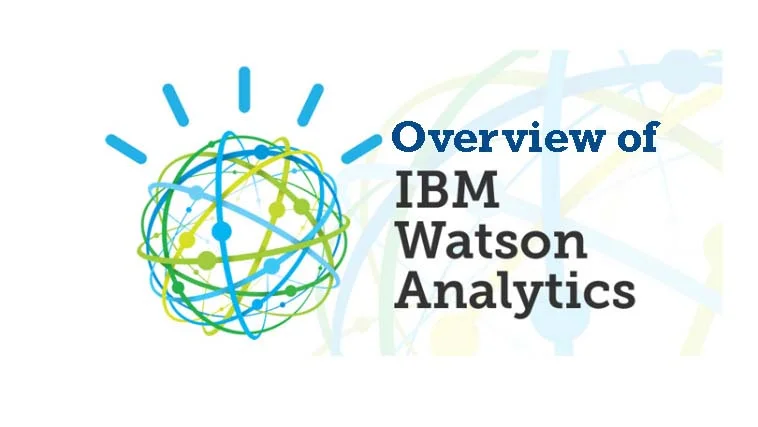
- Overview: IBM’s Watson Analytics is renowned for its cognitive capabilities, combining AI, machine learning, and natural language processing for robust data analysis.
- Features:
- Automated data discovery and pattern recognition.
- Predictive modeling with cognitive analytics.
- Scalable cloud-based platform.
- Use Cases:
- Market trend analysis and customer behavior insights.
- Financial risk assessment and compliance monitoring.
- Why It Stands Out: Watson Analytics brings advanced AI with a user-friendly interface, ideal for organizations that need data interpretation without heavy technical involvement.
DataRobot
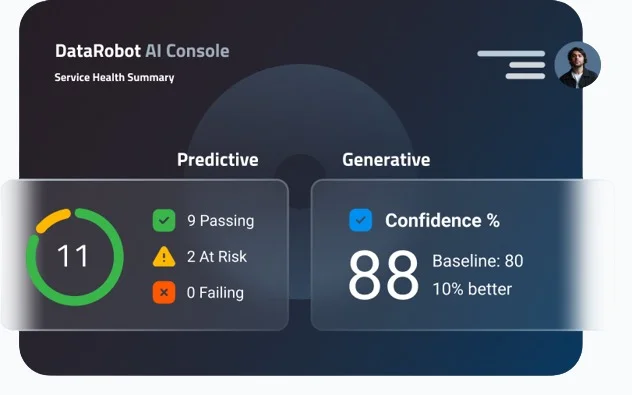
- Overview: DataRobot specializes in automated machine learning, allowing users to build, deploy, and manage AI models without deep expertise in AI or machine learning.
- Features:
- AutoML for quick model development and deployment.
- Real-time prediction and continuous learning.
- Model interpretability and management tools.
- Use Cases:
- Financial forecasting for portfolio management.
- Customer churn analysis for subscription services.
- Why It Stands Out: DataRobot is a comprehensive AI tool designed for speed and ease of use, empowering data scientists and analysts with tools to create models without complex coding.
Amazon SageMaker
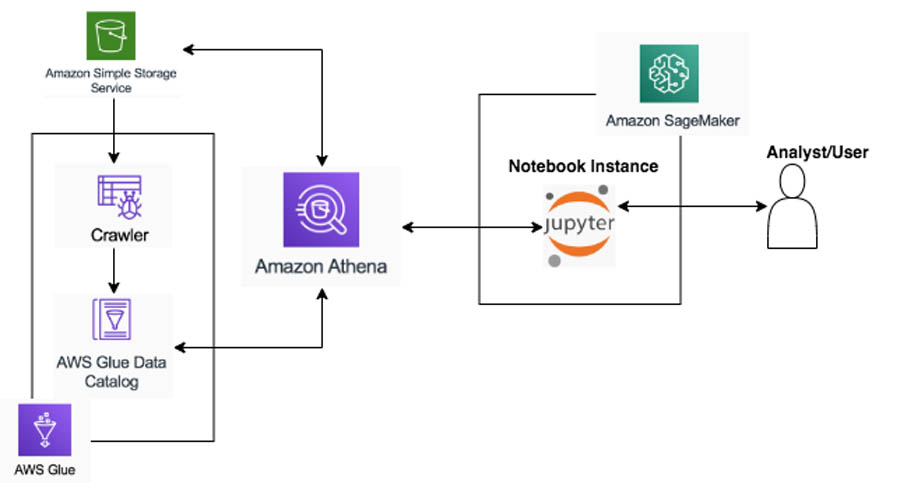
- Overview: Amazon SageMaker is a machine learning platform on AWS, providing an integrated environment for building, training, and deploying ML models.
- Features:
- Managed infrastructure for model building and deployment.
- Built-in algorithms and integration with Amazon’s data ecosystem.
- Real-time predictions with low latency.
- Use Cases:
- Demand forecasting and inventory management in retail.
- Sentiment analysis for social media monitoring.
- Why It Stands Out: With Amazon’s vast data services, SageMaker is a powerful tool for companies already invested in the AWS ecosystem, making it easier to scale AI applications.
RapidMiner
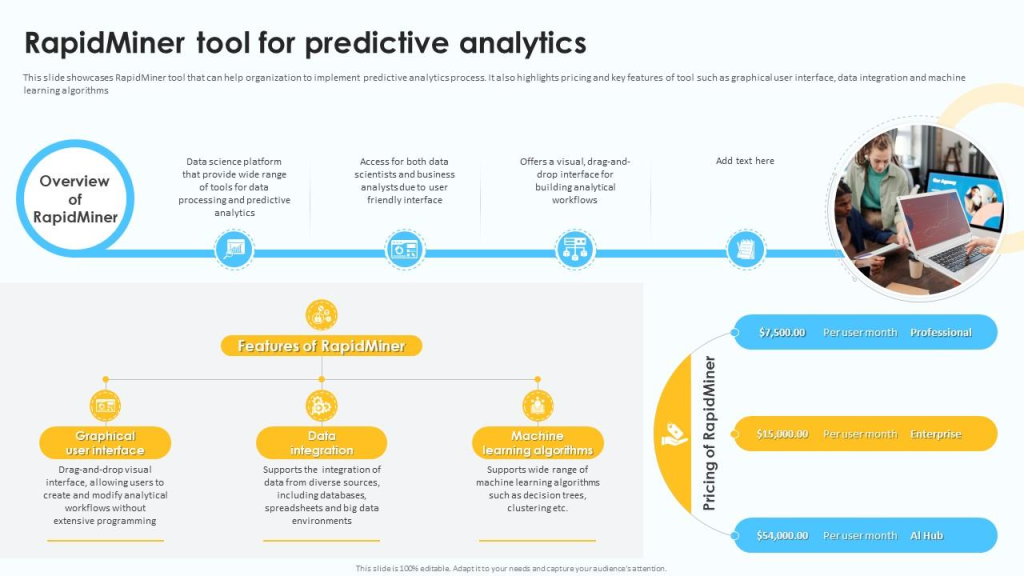
- Overview: RapidMiner provides an end-to-end platform for data science and machine learning workflows, designed for analytics teams.
- Features:
- Automated machine learning with visual workflows.
- Real-time predictive analytics and reporting.
- Robust data integration and transformation tools.
- Use Cases:
- Manufacturing process optimization and predictive maintenance.
- Fraud detection in finance and insurance.
- Why It Stands Out: RapidMiner is suitable for analysts who prefer visual-based workflows, reducing the coding requirements to build powerful machine learning models.
H2O.ai
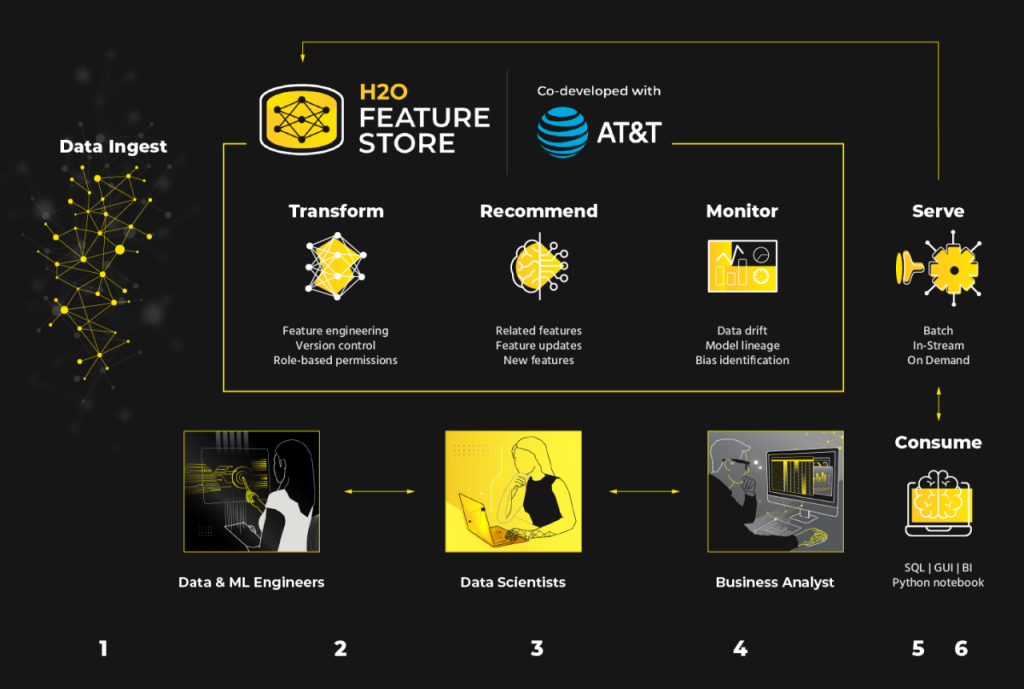
- Overview: H2O.ai is an open-source platform offering automated machine learning and AI solutions, known for its speed and scalability.
- Features:
- AutoML for faster model training.
- Distributed machine learning for big data.
- Flexible deployment options.
- Use Cases:
- Credit risk modeling for financial institutions.
- Real-time analytics in telecommunications.
- Why It Stands Out: With a focus on speed and scalability, H2O.ai is ideal for organizations dealing with big data and requiring fast, actionable insights.
How to Choose the Right AI Tool for Data Analysis?
Selecting the right AI tool depends on several factors, including:
- Scalability Needs: Larger organizations may benefit from platforms like Amazon SageMaker, while smaller teams might prefer Power BI or Tableau.
- Industry Requirements: Specific industries have unique requirements. For instance, finance requires advanced risk management tools, while retail benefits from predictive analytics for demand forecasting.
- User-Friendliness: Some tools, like IBM Watson and Tableau, are designed for ease of use, whereas others, like H2O.ai, require more technical expertise.
- Integration Capabilities: Ensure compatibility with existing data sources and IT infrastructure.
Conclusion
AI tools for data analysis have transformed the way businesses approach data-driven decision-making, making it more efficient and accessible. By choosing the right AI tool, organizations can unlock the full potential of their data, optimize processes, and maintain a competitive edge. From visual analytics to automated machine learning, the tools highlighted here offer robust solutions for every need, from small teams to enterprise-level organizations. Embracing AI in data analysis is not just a trend; it’s an essential step toward sustainable growth in the digital era.