In today’s digital landscape, data has emerged as one of the most valuable assets for organizations. With the vast amounts of information generated daily, the ability to anticipate future trends or outcomes is critical. Predictive analytics allows businesses to leverage this data, combining historical insights and real-time information to forecast future scenarios with a high degree of accuracy.
Through predictive analytics, companies can analyze patterns in vast datasets, identify trends, and make data-backed decisions that enhance strategic planning and operational efficiency. From predicting customer purchasing behaviors to assessing financial risks or identifying potential machinery failures, predictive analytics transforms raw data into actionable insights. By tapping into predictive analytics, companies in virtually every sector are able to not only react to present conditions but also make informed decisions that drive future success. As such, predictive analytics has evolved into an indispensable tool for modern businesses seeking a competitive edge in an information-rich world.
Why Use AI for Predictive Analytics?
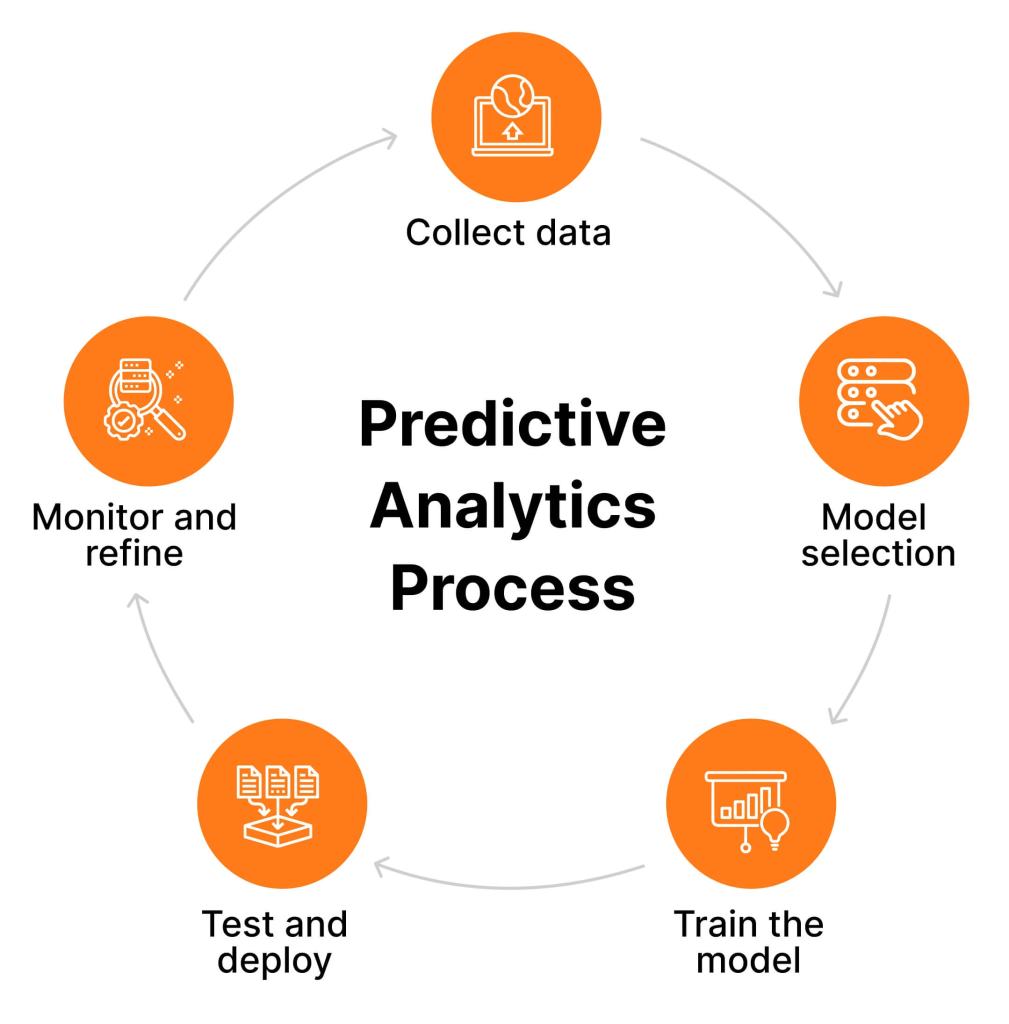
AI technology has revolutionized predictive analytics, enhancing its accuracy, scalability, and accessibility. Here are several reasons why AI is instrumental in taking predictive analytics to the next level:
- Automating Complex Data Processing
Traditional predictive analytics methods often required extensive manual data preparation and model development, which could be time-consuming and prone to human error. AI automates these tasks through machine learning algorithms that can rapidly analyze and process massive datasets. AI-enabled tools manage everything from data cleaning to feature engineering, ensuring faster, more accurate outcomes with minimal human intervention. This automation empowers data analysts and business users to focus more on interpreting insights rather than on repetitive processing tasks. - Enhancing Prediction Accuracy
AI algorithms, especially machine learning models, can uncover complex patterns and relationships within data that traditional statistical methods might miss. Through deep learning models, for example, AI can analyze intricate data structures, handle non-linear relationships, and refine predictions continuously as new data becomes available. This ability to learn and adapt improves the precision of predictions, enabling organizations to make decisions with greater confidence. As a result, companies can rely on AI-enhanced predictive analytics to anticipate customer behavior, market trends, risk factors, and more with a high level of accuracy. - Handling and Integrating Diverse Data Sources
Modern organizations often deal with a variety of data types and formats, from structured databases to unstructured data such as text, images, or social media posts. AI’s versatility allows it to process these diverse data sources, merging information from different channels into a unified model for prediction. Natural Language Processing (NLP) enables AI systems to interpret text data, while image recognition algorithms can process visual data. This capability is invaluable in applications like customer sentiment analysis, where textual and visual data may need to be combined to understand user emotions and preferences fully. - Enabling Scalability Across Massive Datasets
With AI-driven predictive analytics, companies can analyze large datasets without sacrificing speed or accuracy. In industries such as finance, retail, and healthcare, where data volumes can be massive, AI-powered tools handle scalability effortlessly. They process millions of data points quickly, providing insights that would be impossible to achieve with traditional analytical methods alone. This scalability is crucial for enterprises looking to expand their predictive analytics efforts as their data grows, ensuring consistent performance across large-scale implementations. - Producing Granular Insights for Precision Decision-Making
AI-powered predictive analytics tools provide insights at a highly granular level, allowing organizations to drill down into specific aspects of their data. For instance, in customer segmentation, AI can help identify micro-segments based on individual preferences, behaviors, or demographics, enabling highly personalized marketing strategies. Similarly, in predictive maintenance, AI can detect subtle anomalies in machinery data that may indicate a potential failure. These detailed insights support precision decision-making, helping companies optimize operations, reduce costs, and improve customer satisfaction.
Top AI Tools for Predictive Analytics
1. Google Cloud AutoML
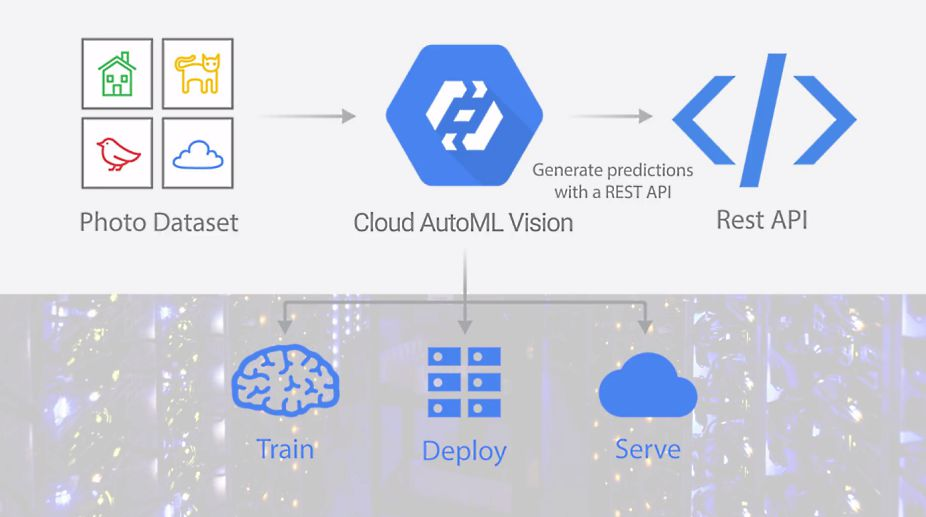
Overview: Google Cloud AutoML is a user-friendly machine learning tool that allows developers and analysts to build predictive models without a strong background in machine learning. The platform automates model training and fine-tuning, making it ideal for organizations of all sizes.
- Features:
- Automated model selection and hyperparameter tuning.
- Integration with Google BigQuery for seamless data processing.
- Supports structured and unstructured data (e.g., images and text).
- Benefits:
- Simplifies the model-building process, ideal for non-experts.
- Cost-effective with a pay-as-you-go model.
- Use Cases: Customer churn prediction, sales forecasting, demand planning.
2. IBM Watson Studio
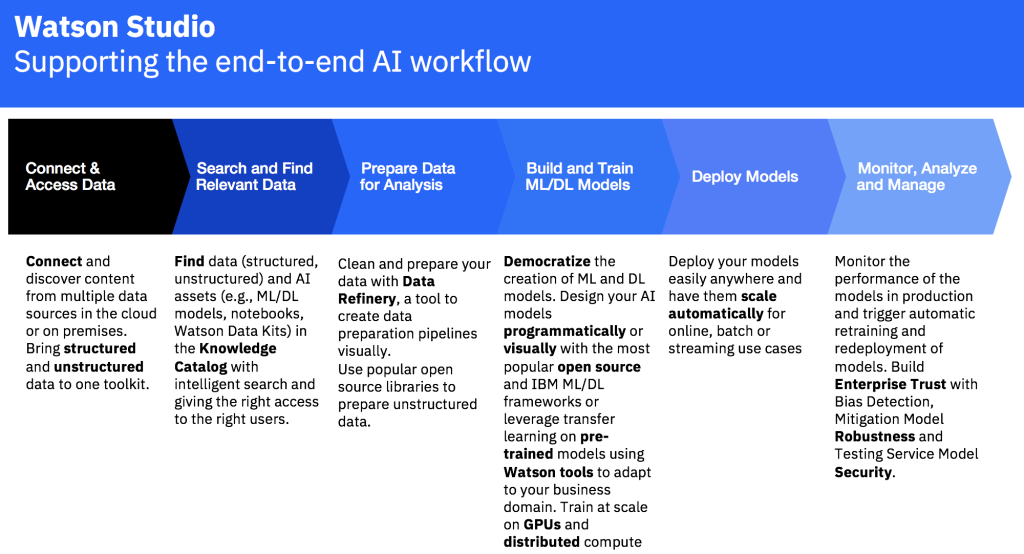
Overview: IBM Watson Studio offers a comprehensive suite for data scientists, enabling collaborative model building, training, and deployment. Its predictive analytics capabilities are powered by AI, offering an extensive library of algorithms and machine learning tools.
- Features:
- Automated model lifecycle management.
- Advanced NLP and machine learning tools.
- Scalable across cloud and on-premises infrastructure.
- Benefits:
- Robust tools for large-scale predictive analytics.
- Flexibility to customize models extensively.
- Use Cases: Healthcare diagnostics, fraud detection, financial forecasting.
3. DataRobot
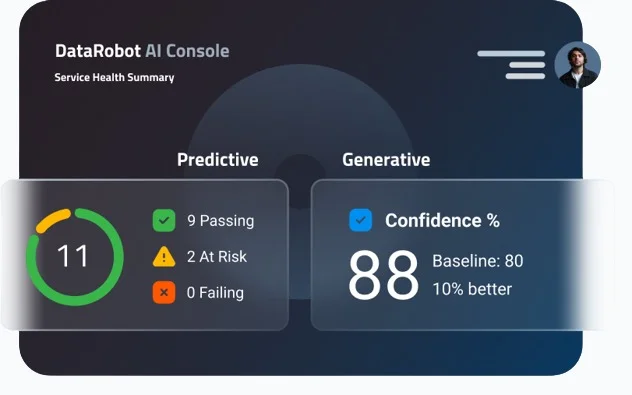
Overview: DataRobot is known for its AutoML capabilities, which are specifically tailored for predictive analytics. It empowers data scientists and business analysts alike with tools to automate data preprocessing, feature engineering, and model selection.
- Features:
- Automated model training, tuning, and deployment.
- Real-time prediction and model monitoring.
- Integration with a wide range of data sources.
- Benefits:
- Accelerates time-to-value for predictive analytics projects.
- Offers interpretability tools to understand model outputs.
- Use Cases: Retail demand forecasting, risk assessment, marketing analytics.
4. H2O.ai
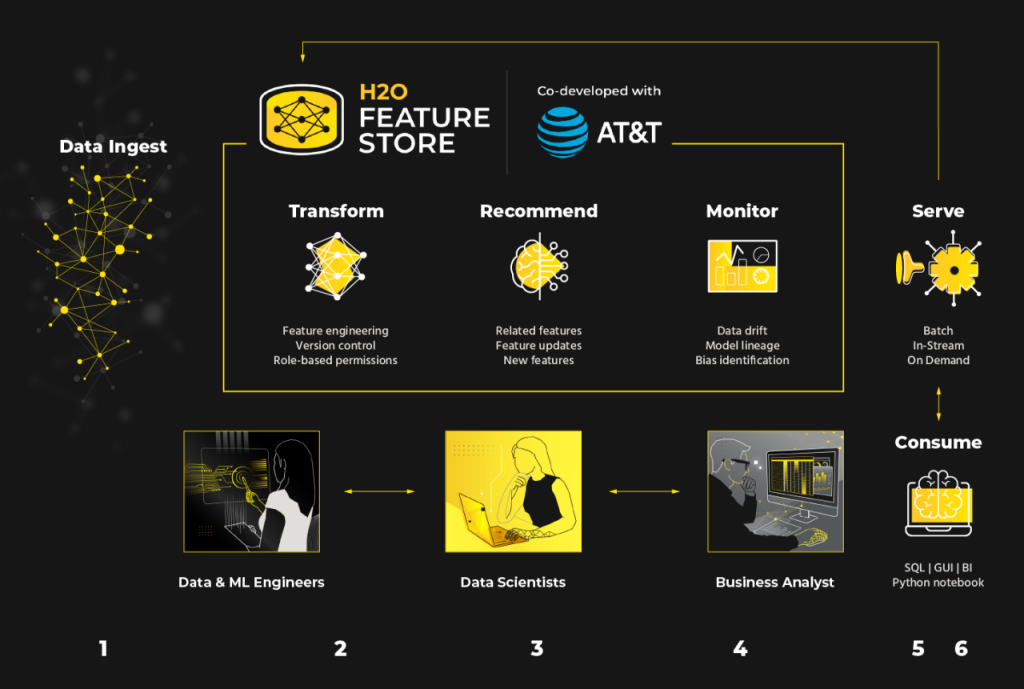
Overview: H2O.ai provides open-source and enterprise versions of its machine learning and predictive analytics platform. Known for its accuracy and efficiency, H2O.ai offers features like AutoML and support for deep learning models, catering to both novices and experts.
- Features:
- Supports a range of machine learning algorithms.
- AutoML functionality for rapid model prototyping.
- Integration with big data frameworks like Apache Spark.
- Benefits:
- High customization and scalability options.
- Cost-effective, especially for open-source users.
- Use Cases: Credit scoring, predictive maintenance, insurance risk analysis.
5. SAS Advanced Analytics
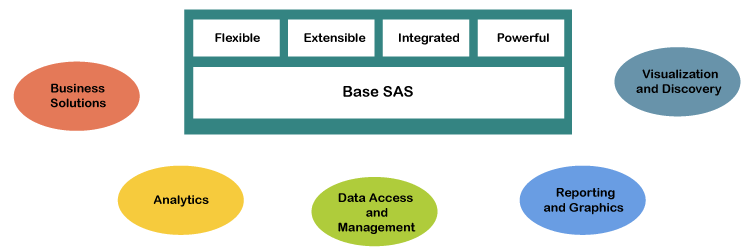
Overview: SAS offers some of the most powerful tools for data analytics and predictive modeling. It’s designed for enterprises needing extensive data manipulation capabilities and advanced statistical modeling.
- Features:
- Comprehensive predictive analytics modules.
- Statistical analysis and optimization.
- Integrations with Hadoop, SAP, and other enterprise systems.
- Benefits:
- Superior statistical modeling capabilities.
- Proven reliability in enterprise environments.
- Use Cases: Banking fraud detection, clinical trials, supply chain forecasting.
6. Azure Machine Learning
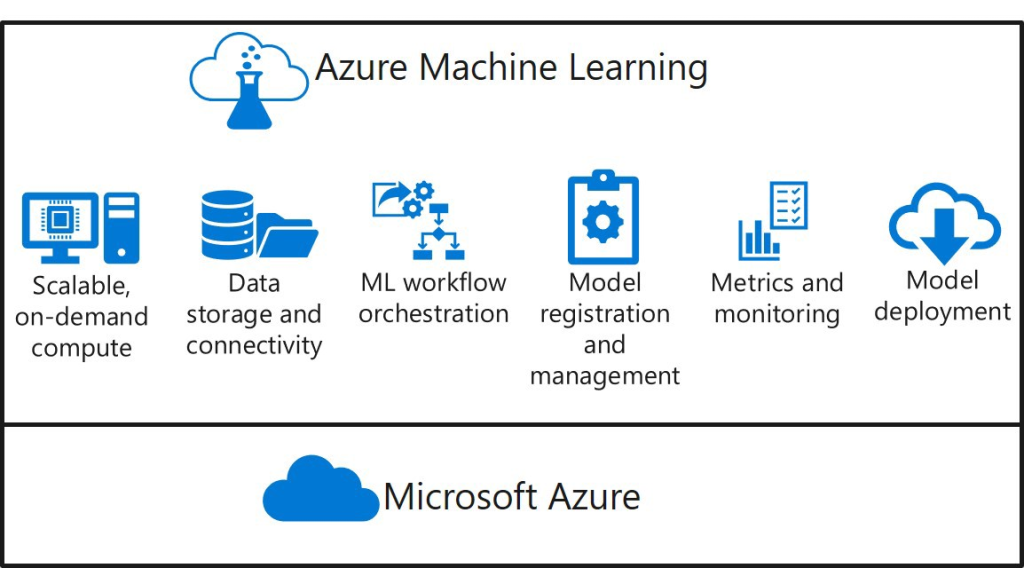
Overview: Azure Machine Learning provides a comprehensive platform for predictive analytics, featuring automated machine learning capabilities, seamless integration with Microsoft Azure’s data services, and scalability for complex projects.
- Features:
- Extensive machine learning model libraries.
- Automated machine learning capabilities.
- Supports Python and R for custom model development.
- Benefits:
- Deep integration with Microsoft’s ecosystem.
- Scalable for small to large enterprises.
- Use Cases: Energy consumption forecasting, predictive maintenance, customer segmentation.
7. RapidMiner
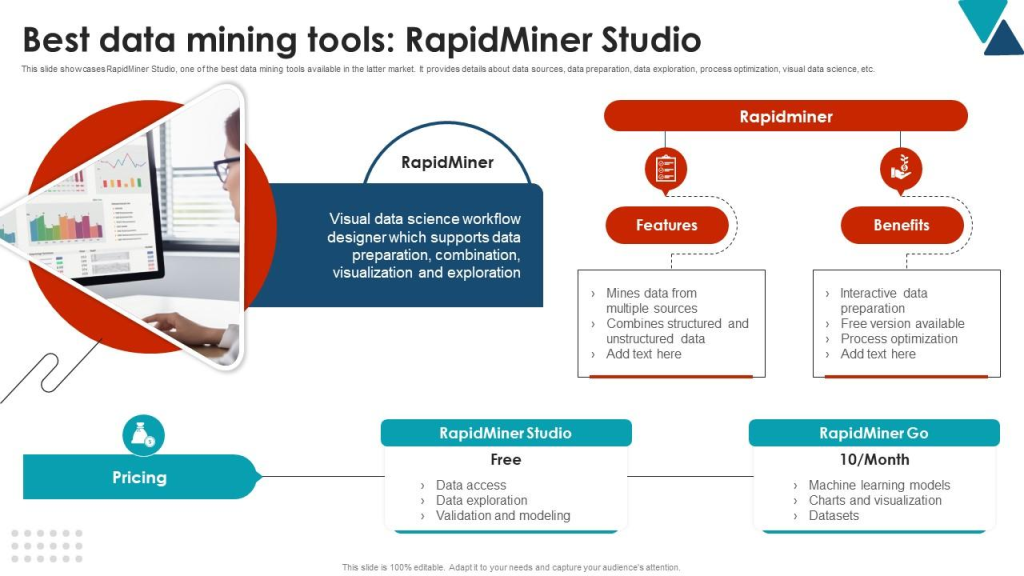
Overview: RapidMiner offers a powerful platform for predictive analytics that supports a wide array of use cases across industries. It provides a no-code/low-code environment ideal for business analysts and data scientists alike.
- Features:
- Drag-and-drop interface for non-technical users.
- Extensive data connectors and ETL functionalities.
- Integrates with popular machine learning frameworks.
- Benefits:
- Accessible for non-programmers.
- Powerful enough for complex predictive models.
- Use Cases: Customer retention, revenue prediction, workforce optimization.